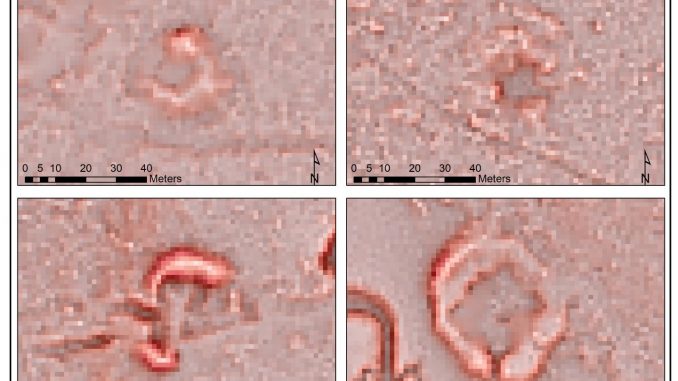
On the Forest where I work, the vegetation from about mid-April until early-November makes pedestrian survey in anything but a plowed field next to impossible. Poison ivy is just slightly more ubiquitous than the thorny blackberry bush in non-cultivated fields and forest edges. LiDAR (Light Detection And Ranging) is a welcome tool.
Jack Nance was one of the prominent archaeologists that worked in the region, so much so that it would probably be irresponsible to write a report to the State Historic Preservation Officer (SHPO) without citing him.
Nance, perhaps drawing on his experiences in the Cumberland River Valley, lamented the difficulty of pedestrian survey in heavy vegetation and remarked how the results of those who tried it were questionable.
So when Lipo, Singer, and Davis (2018) described their object-based image analysis technique for detecting pre-contact mounds in LiDAR, I was a little eager to read the paper in Southeastern Archaeology (June 2018). They also cited Jack Nance a few times in their paper! Their abstract begins:
The study of precontact anthropogenic mounded features—earthen mounds, shell heaps, and shell rings—in the American Southeast is stymied by the spotty distribution of systematic surveys across the region. Many extant, yet unidentified, archaeological mound features continue to evade detection due to the heavily forested canopies that occupy large areas of the region, making pedestrian surveys difficult and preventing aerial observation
The technique they describe is “object-based image analysis” (OBIA) and is run with GIS software in a “semi-automated” manner.
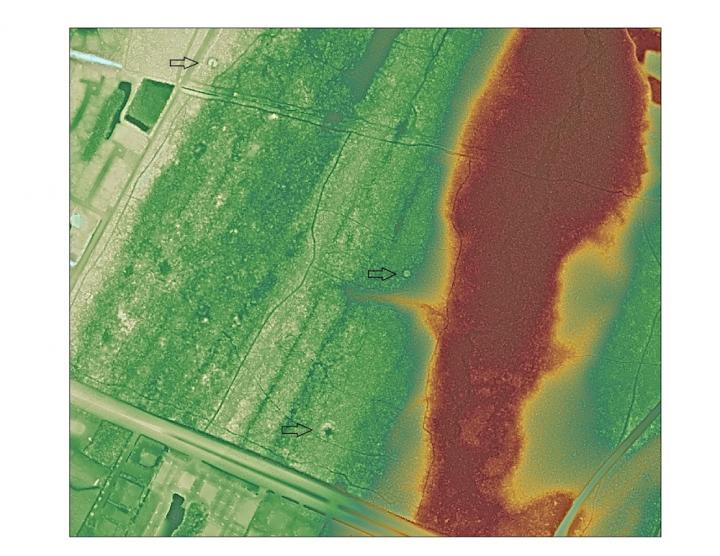
Essentially, the authors used existing LiDAR data, and focused on four rasters based on a Digital Elevation Model (DEM): slope, maximum focal statistics, hillshade, and red-relief image mapping (RRIM). To get the details on the advantages/disadvantages of these rasters, be sure to check out the original paper. Incidentally, it offers a nicely detailed, yet concise explanation of LiDAR.
With these rasters, the authors then created some negative templates to do some template matching. In this part of the workflow, the idea is to begin to eliminate modern and natural features. This continues in the automated portion using eCognition, a software package developed by Trimble which further eliminates false-positives by checking things like asymmetry, compactness, et.
The results are imported into ArcMap and the final bit of manual workflow (checking against USGS maps, etc.) is done.
The results for them was the initial identification of 7,115 sites. The OBIA method allowed them to reduce this to
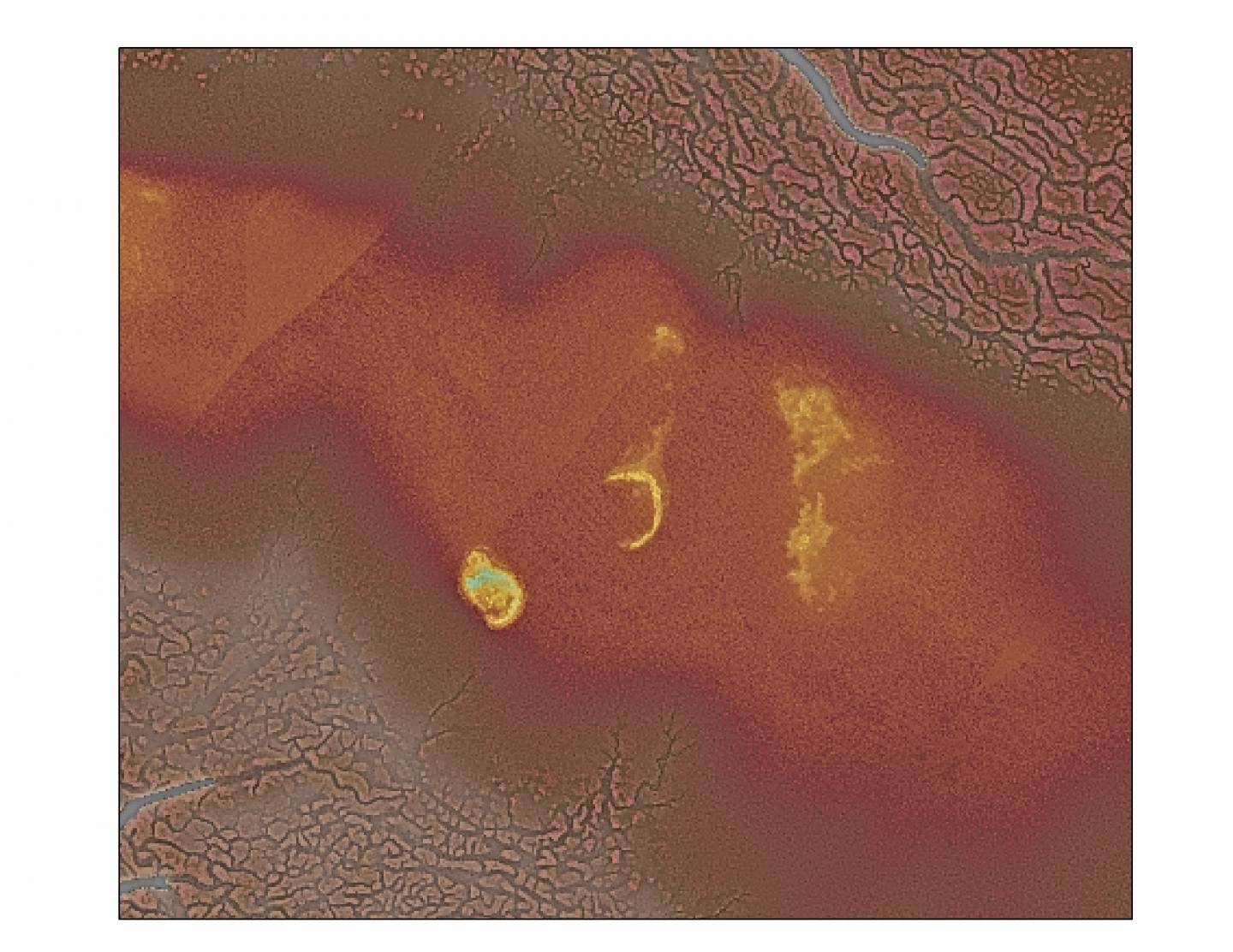
a very manageable 186 sites and then to 15 that were the most promising. Of those 15 sites, 5 were on public lands that
didn’t require a boat to access. Three of these were already documented and so the authors ended up with 2 sites to ground truth.
One ended up being a shell ring about 15m in diameter; the other was a 2m high mound that had evidence of prior looting.
This is definitely an effective workflow and method. I just wonder, however, if there might be some alternative in the “automated” portion that happens in eCognition. The authors probably got the 75% discount, but working for the Forest Service, it would probably be full price for our shop. I’d love to see a video of their overall workflow. Perhaps there are some opportunities to process the asymmetry, circularity, and compactness checks in other software like SAGA, QGIS, etc.
Watched a documentary on forgotten Amazonian civilization last night, LiDAR is amazing.
Yeah, LiDAR is pretty amazing stuff. It’s hard to wrap your head around the idea of so many fired lasers in such a smalls space creating so many data points from the canopy to the last returns at the ground floor. I like playing around with it in our region, but unfortunately the resolution of available LiDAR for our Forest isn’t that great. We do spot some larger features like old roads and the like though.